Artificial Intelligence (AI) has undergone a remarkable evolution, with its origins extending to ancient Greece and reaching pivotal milestones in computing history. Moreover, this blog navigates the definition, history, and diverse applications of AI, unraveling its transformative journey. From Alan Turing’s foundational inquiries to contemporary advancements like ChatGPT’s generative models, the trajectory of AI Reflecting profound shifts, AI has evolved into an integral force, adept at mimicking human cognition and problem-solving over time. Moreover, the narrative delves into the historical tapestry of AI, vividly illustrating its growth from theoretical concepts to practical implementations. This journey showcases its profound impact on both technology and society.
Understanding AI: A Historical Perspective It’s Here
Artificial Intelligence (AI) represents a field that has transformed the landscape of computing by simulating human problem-solving and also decision-making abilities. This exploration delves into the evolution of AI, Bridging from John McCarthy’s comprehensive 2004 definition to the foundational inquiries initiated by Alan Turing in 1950, signifies a pivotal shift in the narrative of artificial intelligence evolution.
John McCarthy’s Vision (2004):
John McCarthy’s 2004 definition stands as a cornerstone, encapsulating the core principles of AI. Emphasizing the fusion of science and engineering, McCarthy envisioned the creation of intelligent machines. This definition serves as a pivotal reference point, consequently enhancing our understanding of the broader landscape of AI.
Alan Turing’s Pioneering Inquiry (1950):
The origins of AI discussions trace back to Alan Turing’s seminal work in 1950. Turing posed a fundamental question that would shape the course of AI history: “Can machines think?” This inquiry not only set the stage for a profound exploration into the capabilities of machines but also laid the groundwork for the renowned Turing Test.
The Turing Test:
Alan Turing’s groundbreaking work introduced the concept of the Turing Test, a landmark assessment designed to evaluate a machine’s ability to exhibit intelligence comparable to human cognition. The test involved a human interrogator attempting to discern between computer-generated and also human responses, marking a crucial milestone in the quest to understand machine intelligence.
AI in McCarthy and Turing’s Era
Examining the historical context, McCarthy and Turing operated in an era where computing technology was in its nascent stages. The conceptualization of AI was rooted in the optimism that machines could emulate human thought processes. The limited computational power of the time, however, presented challenges in realizing these ambitious visions.
Balance Pioneering Potential: Generative Models And Ethics
Early Milestones in AI:
As AI discussions unfolded, significant milestones marked the field’s progress. McCarthy’s definition paved the way for early explorations into AI applications, but it was not until the development of the Logic Theorist by Allen Newell, J.C. Shaw, and also Herbert Simon in 1956 that tangible progress was made. This event signaled the birth of practical AI software.
Neural Networks and Learning Algorithms
The 1980s witnessed a paradigm shift with the introduction of neural networks employing backpropagation algorithms for learning. During this period, the groundwork was laid for AI applications, fostering enhanced learning capabilities and contributing to contemporary AI models.
Artificial Intelligence Today: A Landscape of Possibilities
Deep Learning vs. Machine Learning:
In the contemporary AI landscape, deep learning and machine learning play pivotal roles. Deep learning, as a subset of machine learning, utilizes neural networks with multiple layers to automate feature extraction. Moreover, understanding the distinctions between these two subfields is essential for navigating the diverse applications of AI.
Generative Models and Language Processing:
The rise of generative models, exemplified by GPT-3 and BERT, signifies a revolutionary shift in natural language processing. These models, trained on vast unlabeled datasets, showcase the potential for broad AI adoption, particularly in the realm of language understanding and generation.
Looking Ahead: Ethical Considerations and Challenges Here
Ethical Implications of AI:
As AI continues its exponential growth, ethical considerations become paramount. The implications of AI in business, combined with the emergence of generative models, raise questions about responsible AI use. Ensuring that AI benefits society ethically and responsibly is a key consideration for the future.
AI’s Transformative Potential:
The scalability of foundation models and the potential for frictionless hybrid-cloud environments hint at a transformative future. As generative models redefine the capabilities of AI, consequently, the prospect of efficient, accurate automation across diverse domains becomes increasingly tangible.
Navigating the Complex Tapestry of Artificial Intelligence
Retrospective and Prospective Views:
Reflecting on the journey from McCarthy’s vision to Turing’s inquiries and the present-day landscape of AI, it becomes evident that the field is dynamic and continually evolving. The intersection of technological advancements, ethical considerations, and transformative potential shapes the intricate tapestry of AI.
Balancing Innovation with Responsibility:
As AI pioneers forward, the challenge lies in balancing innovation with responsibility. Furthermore, ethical considerations must guide the deployment of AI technologies, ensuring that the transformative power contributes positively to society and enterprise.
The history of AI is a narrative of curiosity, inquiry, and innovation, with each chapter contributing to the rich legacy of artificial intelligence. The ongoing evolution of AI holds the promise of reshaping our world, presenting both unprecedented opportunities and challenges on the horizon.
Evolution of AI Definitions:
Stuart Russell and Peter Norvig, in their influential work “Artificial Intelligence: A Modern Approach,” categorized AI into systems that think or act like humans and those that think or act rationally. This classification laid the foundation for understanding AI goals, distinguishing between human-centered approaches and rational problem-solving.
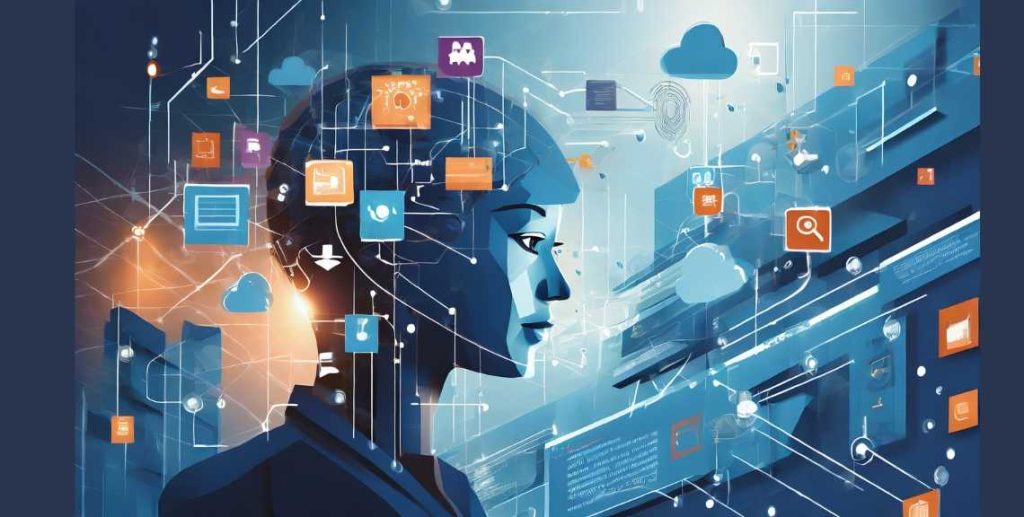
Decoding Artificial Intelligence: Weak AI vs. Strong AI
Distinguishing between Weak AI and Strong AI, the former, also known as Narrow AI, focuses on specific tasks like virtual assistants and autonomous vehicles. Strong AI, encompassing Artificial General Intelligence (AGI) and Artificial Super Intelligence (ASI), remains theoretical, aspiring to achieve human-like consciousness and surpass human intelligence.
Deep Learning vs. Machine Learning:
Within AI, deep learning and machine learning play integral roles. Deep learning, a subset of machine learning, involves neural networks with more than three layers, automating feature extraction and enabling scalability. Understanding the nuances between deep learning and classical machine learning helps grasp their distinct learning approaches and applications.
The Rise of Generative Models:
Generative AI, exemplified by models like GPT-3 and BERT, marks a paradigm shift, particularly in natural language processing. These models, trained on vast unlabeled datasets, showcase the potential for broad AI adoption. Foundation models, with minimal fine-tuning, promise efficient, accurate automation across diverse domains.
Tremendous AI In Action: Real-world Transformations
Real-world applications of AI abound, ranging from speech recognition and customer service automation to computer vision, recommendation engines, and automated stock trading. Each application showcases how AI is transforming industries, enhancing user experiences, and optimizing complex processes.
- Speech Recognition: AI pioneers speech recognition, employing Natural Language Processing (NLP) to convert spoken words into text. Integrated into mobile devices and virtual assistants like Siri, this technology transforms human-computer interaction, streamlining communication.
- Customer Service Automation: AI-driven virtual agents reshape customer service, automating interactions along the customer journey. From answering FAQs to providing personalized advice, these agents enhance user engagement on websites, social media, and messaging platforms. Examples include messaging bots on e-commerce sites, messaging apps like Slack, and voice assistants like Amazon’s Alexa.
- Computer Vision: AI’s prowess in computer vision interprets and extracts meaningful information from digital images and videos. Applications range from social media photo tagging to healthcare radiology imaging and also the evolution of self-driving cars, showcasing diverse impacts on industries.
- Recommendation Engines: AI algorithms drive recommendation engines, analyzing past consumption behavior to identify data trends. Seen prominently in e-commerce platforms, these engines optimize the shopping experience by offering relevant add-on recommendations during the checkout process.
- Automated Stock Trading: In the realm of finance, AI makes significant strides through the utilization of automated stock trading platforms, employing high-frequency trading algorithms. By executing thousands or millions of trades daily, these systems effectively leverage AI’s analytical capabilities for optimal portfolio management and dynamic responses to market fluctuations.
- Transformation Across Industries: AI’s broad impact across industries transforms traditional processes and fuels innovation. From improving customer interactions to enhancing visual perception and optimizing financial strategies, AI emerges as a driving force shaping the future of technology and business.
AI Unveiled: A Timeline of Revolutionary Breakthroughs
History of Artificial Intelligence:
A chronological journey through key dates and names in the history of AI provides insights into its evolution. From Alan Turing’s seminal work to IBM’s Deep Blue defeating chess champion Garry Kasparov and recent breakthroughs like generative models, each milestone contributes to the rich tapestry of AI development.
Artificial intelligence (AI) has come a long way since its inception in the 1940s. Over the years, we’ve witnessed remarkable advancements in machine learning, natural language processing, computer vision, and other AI fields. Consequently, AI’s progress birthed applications reshaping our lives in unimaginable ways, surpassing conventional expectations and boundaries.
Embarking on a Timeline: Unveiling Key Dates and Names
- 1943: Warren McCulloch and Walter Pitts developed the first artificial neuron, laying the foundation for neural networks.
- 1950: Alan Turing introduces the Turing Test, a benchmark for machine intelligence.
- 1956: The Dartmouth Conference, organized by John McCarthy, Marvin Minsky, Claude Shannon, and Nathaniel Rochester, is held, marking the official birth of AI as a field of study.
- 1966: The first AI chatbot, ELIZA, is developed by Joseph Weizenbaum.
- 1969: Marvin Minsky and Seymour Papert publish their book “Perceptrons,” which raises concerns about the limitations of AI.
- 1972: The first expert system, MYCIN, was developed by Edward Shortliffe and others at Stanford University.
- 1979: The first successful voice recognition system, developed by Raj Reddy at Carnegie Mellon University, is demonstrated.
- 1980: Backpropagation, a crucial algorithm for training artificial neural networks, is rediscovered.
- 1982: Rule-based AI systems, such as R1/XCON, successfully configure computers.
- 1997: IBM’s Deep Blue computer defeats world chess champion Garry Kasparov, a landmark achievement for AI.
- 1997: The term “Deep Learning” is coined by Geoffrey Hinton, Yoshua Bengio, and Yann LeCun.
- 2001: The Roomba, a robotic vacuum cleaner, becomes a commercial success, demonstrating the potential of AI in consumer products.
- 2005: The first self-driving car, created by Stanford University’s Stanley team, wins the DARPA Grand Challenge.
- 2012: AlexNet triumphs in ImageNet, a computer vision breakthrough.
- 2014: OpenAI, a non-profit research company dedicated to developing safe and beneficial artificial general intelligence, is founded.
- 2016: Google DeepMind’s AlphaGo program defeats world champion Go player Lee Sedol, a significant milestone for AI.
- 2022: Generative AI models, such as DALL-E 2 and Imagen, generate realistic images and text, demonstrating the power of AI to create.
- 2023: AI continues to evolve at a rapid pace, impacting various industries, including healthcare, finance, transportation, and manufacturing.
Transformative Future: AI Ethics Amid Model Growth
As AI continues to advance, ethical considerations become paramount. AI’s evolution in business, alongside generative model growth, prompts questions on responsible AI application and usage. Frictionless hybrid-cloud potential and also foundation model scalability point towards a transformative future in AI development.
The rapid advancement of artificial intelligence (AI) brings a wave of exciting opportunities and transformative potential. Still, it also raises critical ethical considerations and challenges that must be carefully evaluated to ensure responsible and beneficial AI development and deployment.
Ethical Implications of AI in Business: The AI Evolution in business operations raises various ethical concerns, especially concerning the use of data, algorithms, and decision-making processes. As AI seamlessly integrates into business operations, it’s vital to proactively guard against biases, discrimination, and also privacy breaches.
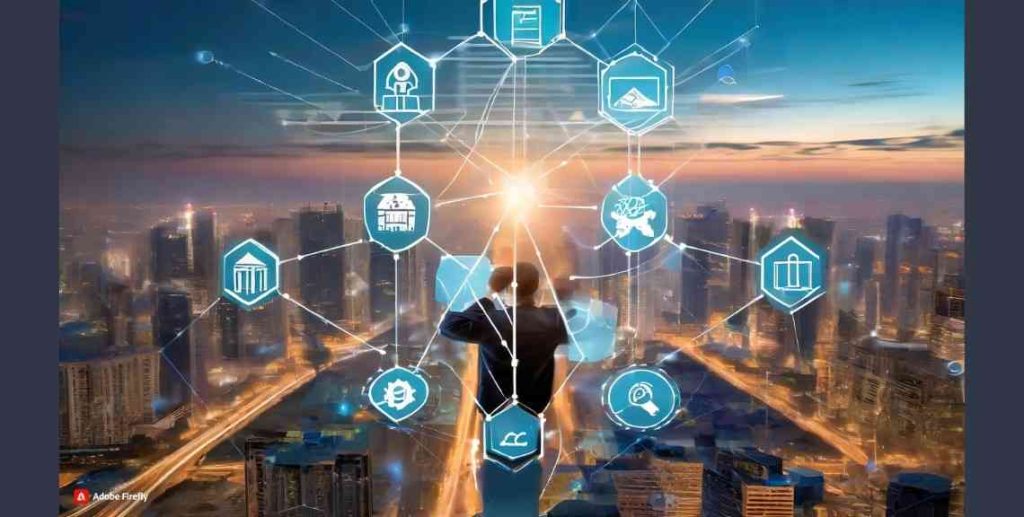
- Data Bias: AI algorithms are trained on vast amounts of data; consequently, if this data contains inherent biases, those biases can be amplified, perpetuating discrimination.
- Algorithmic Accountability: The complexities of AI algorithms can make it difficult to understand and explain their decision-making processes.In the AI evolution, the lack of transparency not only raises concerns about accountability and fairness but also challenges understanding.
- Privacy Protection: Adhering to stringent privacy regulations is crucial when collecting, storing, and analyzing personal data for AI.
Generative Models and Responsible AI Use
Generative models, a facet of AI, hold transformative potential by creating diverse content like images and text. However, their ability to generate realistic and also even deceptive content raises concerns about misinformation, manipulation, and potential misuse.
- Misinformation and Deepfakes: Generative models can be used to create fake videos, and audio recordings, Moreover, generating videos and text indistinguishable from real content poses a significant threat to misinformation and manipulation.
- Copyright and Originality: Generative models can produce content that is similar to or even indistinguishable from existing copyrighted material, raising questions about intellectual property and originality.
- Ethics of Creative Content Generation: As generative models become more sophisticated in producing creative content, there is a need to establish ethical guidelines for their responsible use, considering factors such as evaluation, originality, ownership, and potential harm.
- Frictionless Hybrid-Cloud Environments and Scalability: Frictionless hybrid-cloud environments are emerging, promising a transformative impact on the future of AI. Concurrently, the scalability of foundation models brings immense promise to the evolving landscape of AI.
- Frictionless Hybrid-Cloud Environments: Hybrid cloud environments combine on-premises infrastructure with cloud resources, enabling seamless data mobility and workload portability. This level of flexibility not only enhances operational efficiency but also significantly accelerates the development and deployment of AI.
Regarding scalability, foundation models, such as large language models, demonstrate impressive capabilities in tasks like language processing, translation, and code generation. This scalability, consequently, facilitates the development of increasingly powerful AI applications, marking a significant stride in innovation.
Delighted Transformative Future Of Artificial Intelligence
Responsible AI practices converge with advancements in generative models. Additionally, frictionless hybrid-cloud environments’ potential, along with scalable foundation models, collectively signals a transformative future for AI. However, Approaching the future with caution is crucial. Addressing ethical considerations is imperative. Ensuring ethical AI development unlocks its transformative potential for prosperity.
Conclusion: Artificial Intelligence has come a long way from its inception, evolving through defining moments and also technological breakthroughs. The journey from Turing’s question to the era of generative models exemplifies the dynamic nature of AI. As we navigate the future of AI, ethical considerations and responsible deployment will shape its impact on society and enterprise.
Keywords: Artificial Intelligence (AI) | Evolution of AI | Generative Models | ChatGPT | History of AI | Neural Networks | Deep Learning | Machine Learning | Hybrid-Cloud Environments